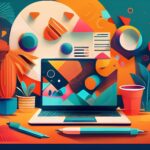
How to Use AI Marketing Tools Effectively
February 2, 2023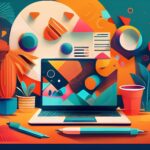
14 Digital Marketing Strategies for E-commerce 2023
February 7, 2023Artificial intelligence (AI) and machine learning (ML) have become increasingly prevalent in the marketing world in recent years. These cutting-edge technologies are revolutionizing how companies approach performance marketing, making it more data-driven and automated.
One of the key benefits of AI and ML in performance marketing is their ability to analyze large amounts of data in real time, providing marketers with valuable insights into consumer behavior and enabling them to make informed decisions. This can lead to increased conversion rates and better ROI, as marketers can tailor their campaigns to their target audience’s specific needs and preferences.
Another advantage of AI and ML is that they can automate many manual, time-consuming tasks involved in performance marketing, freeing up time for marketers to focus on other essential tasks. For example, AI can automate bid optimization and ad placement tasks, allowing marketers to focus on developing more effective campaigns.
AI and ML also allow for greater personalization in performance marketing, as these technologies can analyze consumer data to deliver tailored advertisements and offers. This leads to increased engagement and conversion rates, as consumers are more likely to respond to offers relevant to their interests and needs.
Despite the many benefits of AI and ML in performance marketing, it is essential to remember that these technologies are only as effective as the data they are fed. Marketers must ensure that their data is accurate, up-to-date, and relevant to their target audience. They must also be aware of privacy concerns and take steps to protect consumer data.
In conclusion, using AI and ML in performance marketing is a powerful tool that can help marketers make more informed decisions, automate time-consuming tasks, and deliver highly personalized advertisements. However, using these technologies ethically and responsibly is essential, considering privacy concerns and data quality.