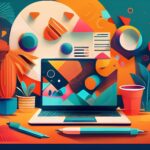
Crafting Compelling Lead Magnets: Turbocharging Your B2B Lead Generation Strategy
September 14, 2023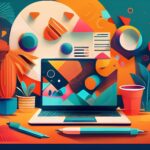
Critical Legal and Compliance Considerations in B2B Lead Generation: Navigating the Landscape Safely
September 15, 2023What is Customer Churn?
Customer churn, often referred to as customer attrition, is the percentage of customers who stop using your products or services during a certain time frame. It’s a critical metric for any business as it directly impacts revenue and growth.
Imagine this: You run a quaint ice cream parlor in a sunny seaside town. At the beginning of the month, you have 100 loyal customers who can’t resist your delicious ice creams. But by the end of the month, you notice that 10 of them haven’t visited in a while. These 10 customers are part of your churn rate. In simple terms, churn is like ice cream melting away – it’s inevitable, but you want to slow it down.
The Impact of Churn on Your Business
Churn can have a domino effect on your business. When customers leave, you not only lose their current revenue but also the potential revenue they would have generated in the future. Moreover, the costs of acquiring new customers to replace the lost ones can be substantial.
Continuing with our ice cream parlor example, let’s see how churn affects the bottom line. Each customer spends an average of $10 per visit, so those 10 customers you lost represent $100 in monthly revenue. Over a year, that’s $1,200. Plus, these customers might have recommended your ice cream to their friends, so you’re losing out on potential referrals too.
Now, you need to invest in marketing to attract new customers. Advertising, promotions, and discounts can add up. It might cost you $300 to acquire a new customer. To replace the churned customers, you’ll need to spend $3,000 ($300 x 10). That’s a net loss of $1,800 ($1,200 – $3,000) for the year. Churn isn’t just losing customers; it’s a financial setback.
Common Causes of Customer Churn
Customer churn can be caused by various factors, including poor customer service, product dissatisfaction, and competitive offers. Let’s explore these causes in detail:
-
Poor Customer Service: Unresponsive customer support and unresolved issues can drive customers away. Imagine your ice cream parlor has rude staff or consistently gets orders wrong. Customers will look for friendlier places to satisfy their sweet cravings.
-
Product Dissatisfaction: If your product doesn’t meet customer expectations, they’re likely to churn. Let’s say your ice cream starts tasting bland or the variety becomes limited. Customers will seek tastier options elsewhere.
-
Competitive Offers: Customers might be enticed by better offers from your competitors. If a new ice cream parlor opens up down the street and offers unique flavors at lower prices, your customers might jump ship.
Real-life Example: Netflix vs. Blockbuster
Remember Blockbuster? At its peak, it was the go-to place for renting movies. However, they didn’t anticipate the digital revolution. Netflix, on the other hand, embraced it. They used customer data to recommend personalized content, keeping subscribers engaged. Blockbuster failed to adapt, leading to its demise.
Netflix is a classic example of understanding and tackling churn. They leveraged data to analyze what movies and TV shows you liked, and they suggested similar content. This personalized approach made you feel like Netflix understood your preferences, keeping you hooked. Blockbuster, however, relied on a one-size-fits-all model. They didn’t see the importance of data and personalized recommendations until it was too late.
In summary, understanding customer churn is like recognizing leaks in your ice cream parlor’s roof. Once you spot them, you can start fixing them, ensuring that your business stays dry and prosperous.
Why AI Marketing Matters
The Evolution of Marketing
Marketing has come a long way from traditional billboards and TV ads. It’s now a data-driven, highly personalized endeavor.
Picture this: In the 1960s, marketing was like a blindfolded game of darts. Marketers would throw their messages into the dark, hoping they’d hit the target. You’d see ads on TV, hear them on the radio, and receive promotional mail in your mailbox. But most of these messages were generic – they didn’t consider who you were, what you liked, or what you needed.
Fast forward to today, and marketing has become a precision art. It’s more like a well-aimed sniper shot, hitting the bullseye every time. How did this transformation happen? AI played a pivotal role.
The Role of Artificial Intelligence
AI marketing leverages machine learning algorithms to analyze vast amounts of data and make real-time decisions. It’s like having a marketing genius with superhuman capabilities.
Consider the data generated daily. Every online click, social media interaction, and purchase leaves a digital footprint. AI can process this data at lightning speed, identifying patterns and trends that humans might miss. For instance, if you often search for hiking gear online, AI can deduce that you’re an outdoor enthusiast. It can then recommend hiking boots when you visit an e-commerce site. This level of personalization makes you more likely to make a purchase.
AI doesn’t stop at data analysis; it also automates tasks. Imagine sending personalized emails to thousands of customers – a Herculean task for a human. AI can do it effortlessly. It can even schedule these emails at the optimal time for each recipient, increasing the chances of engagement.
Benefits of AI Marketing
Why should you care about AI marketing? Here are some compelling reasons:
-
Hyper-Personalization: AI can tailor marketing messages to individual preferences. It’s like having a personal shopper who knows your style perfectly.
-
Predictive Analytics: It can forecast customer behavior, including churn. If AI predicts that a customer is likely to churn, it can trigger automated retention strategies.
-
Efficiency: Automation reduces manual work and human error. This means fewer hours spent on routine tasks and more time for strategic planning.
-
Cost-Effective: AI marketing delivers better ROI. When your marketing efforts are highly targeted, you get more bang for your buck.
Case Study: Amazon’s Personalized Recommendations
Ever wondered how Amazon knows what you want before you do? They use AI to analyze your browsing and purchasing history to recommend products. It’s eerily accurate and keeps customers coming back for more.
Let’s say you’re browsing for a new camera. You look at a few models, read some reviews, but you’re not quite ready to buy. You leave the site, and later, when you’re scrolling through your social media feed, you see an ad for the exact camera you were considering. It’s not a coincidence; it’s AI in action.
Amazon’s recommendation engine analyzes your behavior and compares it to millions of other users. It then predicts what products you’re likely to buy next. This level of personalization keeps you engaged with the platform, increasing the chances of making a purchase.
In summary, AI marketing is like having a marketing team with superhuman abilities. It processes data, automates tasks, and delivers hyper-personalized messages, all of which are instrumental in mastering customer churn prediction.
Data: The Fuel for Churn Prediction
The Data Goldmine
Your business is sitting on a goldmine of data. Every interaction, purchase, and click leaves a digital footprint that can be used to predict churn.
Think of your data as puzzle pieces scattered on a table. Each piece represents a customer’s action or behavior – a click on your website, a purchase, a review, a customer service chat, or even a simple “like” on social media. These pieces may seem disconnected, but when you put them together, they reveal a clear picture of your customers’ journey.
Types of Data Used in Churn Prediction
Churn prediction relies on various data types, including:
-
Behavioral Data: How customers interact with your product. This includes website visits, app usage, and the features they engage with. For instance, if you run a streaming service, it’s essential to know which shows or movies a user watches and how frequently.
-
Demographic Data: Age, location, income – all play a role. Demographic data provides context to behavioral data. It helps you understand if certain customer segments are more likely to churn than others. For example, younger customers might have different preferences than older ones.
-
Transactional Data: Purchase history and spending habits. If you run an e-commerce site, knowing what customers buy, when they buy, and how much they spend is vital. It can reveal patterns that indicate potential churn.
-
Feedback and Surveys: Direct customer feedback is invaluable. This includes product reviews, surveys, and customer support interactions. If a customer leaves a negative review or complains about an issue, it’s a red flag for potential churn.
Consider an online clothing store as an example. Behavioral data might show that a customer used to browse the website daily but has recently stopped. Demographic data might reveal that this customer is in a younger age bracket. Transactional data could indicate that they used to make frequent purchases but haven’t bought anything in a while. Lastly, feedback data might include a customer support ticket where they expressed dissatisfaction with recent purchases. When you put all this data together, it paints a clear picture of a customer who might be on the brink of churning.
The Importance of Data Quality
Garbage in, garbage out. Inaccurate or incomplete data can lead to flawed predictions. Clean, reliable data is essential for churn prediction models.
Think of your data as the ingredients for a gourmet dish. If you use fresh, high-quality ingredients, you’re more likely to create a delicious meal. But if your ingredients are stale or contaminated, your dish will be unappetizing.
To ensure data quality, consider the following:
-
Data Collection Practices: Use reliable methods to gather data. This includes using secure and accurate tools for data collection.
-
Data Cleaning: Regularly clean and preprocess your data. Remove duplicates, correct errors, and handle missing values appropriately.
-
Data Storage: Ensure data is stored securely. Data breaches can not only harm your customers but also erode their trust in your business.
-
Data Governance: Implement data governance policies and procedures to maintain data quality over time.
Data Security Concerns in Churn Prediction
Collecting and storing customer data comes with responsibilities. Ensure you comply with data protection regulations and invest in robust cybersecurity.
Imagine your data as a treasure vault. Inside, you have valuable information about your customers. However, this vault is a prime target for cybercriminals. They want to break in and steal this information for malicious purposes.
To protect your data and, by extension, your customers, follow these best practices:
-
Data Encryption: Use encryption to secure data both in transit and at rest. This ensures that even if a hacker intercepts your data, it remains unreadable.
-
Access Control: Limit access to sensitive data. Only authorized personnel should be able to view or modify it.
-
Regular Audits: Conduct regular security audits to identify vulnerabilities and address them promptly.
-
Compliance: Stay informed about data protection laws in your region and industry. Ensure your practices align with these regulations.
-
Data Minimization: Collect only the data you need for churn prediction. The less data you have, the lower the risk if a breach occurs.
In conclusion, data is the foundation of churn prediction. It’s like the raw material for a masterpiece. To create an accurate and reliable churn prediction model, you need high-quality data. But remember, with great data comes great responsibility – protect it like you would your most valuable assets.
Machine Learning Magic
Introduction to Machine Learning
Machine learning is the backbone of churn prediction. It’s the process of training algorithms to learn from data and make predictions or decisions without being explicitly programmed.
Imagine you have a skilled apprentice in your ice cream parlor. This apprentice doesn’t just scoop ice cream; they learn from every customer interaction. If a customer prefers a particular flavor or always asks for an extra topping, the apprentice remembers. Over time, they become experts at predicting what each customer will order, almost like reading minds.
Machine learning operates similarly. It learns from historical data to make predictions about future events, such as which customers are likely to churn. Instead of hardcoding rules, you feed the algorithm data, and it discovers patterns on its own.
Supervised vs. Unsupervised Learning
In supervised learning, models are trained on labeled data, while unsupervised learning deals with unlabeled data. Churn prediction typically falls under supervised learning.
Think of supervised learning as a teacher guiding a student. The teacher provides the student with examples (data) and tells them what each example represents (labels). The student learns to make predictions based on this guidance. In churn prediction, the labeled data would indicate which customers churned and which didn’t.
Unsupervised learning, on the other hand, is more like a student exploring a library without any guidance. They don’t have labels to tell them what each book is about, but they can still discover patterns and group similar books together. Unsupervised learning can be useful for exploring data and finding hidden insights, although it’s less common in churn prediction.
Building a Churn Prediction Model
Creating a churn prediction model involves several steps:
- Data Collection: Gather relevant data from various sources. In our ice cream parlor, this could include purchase history, customer reviews, and social media interactions. The more data, the better the model’s accuracy.
- Data Preprocessing: Clean and prepare the data for analysis. Imagine you have a stack of handwritten ice cream orders. Some are smudged or contain typos. Data preprocessing is like straightening out these orders, making them readable and consistent.
- Feature Selection: Choose the most relevant variables. Not all data is equally important. For churn prediction, you might focus on variables like purchase frequency, customer satisfaction ratings, and recent interactions.
- Model Selection: Pick the right algorithm for your data. Machine learning offers a variety of algorithms, each with its strengths and weaknesses. Common choices for churn prediction include decision trees, logistic regression, and neural networks.
- Training and Evaluation: Train the model on a portion of your data and evaluate its performance on another portion. This helps you understand how well the model predicts churn and minimizes false alarms.
Imagine this as a training session for your apprentice. You give them a set of past customer interactions (data) and tell them which customers churned and which didn’t (labels). They practice making predictions until they get really good at it. Then, you give them a new set of interactions to see how well they’ve learned.
Evaluation Metrics: How Well Does Your Model Perform?
To gauge the effectiveness of your churn prediction model, you’ll use metrics like accuracy, precision, recall, and F1 score. These help you understand how well your model predicts churn and minimizes false alarms.
-
Accuracy: This metric measures the overall correctness of your model’s predictions. It’s like grading your apprentice on how often they get orders right. However, it can be misleading if your data is imbalanced, meaning you have many more non-churned customers than churned ones.
-
Precision: Precision measures the accuracy of positive predictions. It answers the question: “Of all the customers the model predicted would churn, how many actually did?” A high precision score means your model is good at avoiding false alarms.
-
Recall: Recall measures how many of the actual churned customers your model correctly identified. It answers the question: “Of all the customers who actually churned, how many did the model catch?” A high recall score indicates your model is good at catching churn.
-
F1 Score: The F1 score is a balance between precision and recall. It’s useful when you want to strike a balance between reducing false alarms and catching actual churn.
In our ice cream parlor, imagine you’re testing your apprentice’s accuracy by asking them to predict the next order for a set of customers. If they get most orders right, they have high accuracy. If they consistently predict extra toppings when customers don’t want them (false alarms), their precision is low. If they miss orders that include extra toppings (missed churn), their recall is low. The F1 score helps you find the sweet spot between getting orders right and avoiding mistakes.
In summary, machine learning is the magic wand in your churn prediction toolkit. It transforms raw data into actionable insights, helping you identify and retain customers who are at risk of churning. It’s like having a brilliant apprentice who learns from data and becomes an expert at predicting customer behavior.
Implementing AI Marketing for Churn Prediction
Integration with Customer Relationship Management (CRM)
Your CRM system is a treasure trove of customer data. Integrating AI marketing with CRM can supercharge your churn prediction efforts.
Imagine your CRM system as a master ledger of all your customers. It contains their contact information, purchase history, and interactions with your business. Now, imagine AI marketing as a skilled detective who can extract valuable insights from this ledger.
Integrating AI marketing with CRM is like giving that detective access to your entire case file. It enables your marketing team to create highly targeted campaigns based on individual customer profiles.
Let’s say a customer, Sarah, frequently visits your ice cream parlor and has a preference for exotic flavors. By integrating AI marketing with CRM, you can send her personalized offers, such as a discount on a new exotic flavor that’s just arrived. This level of personalization can significantly reduce Sarah’s likelihood of churning.
Automating Customer Engagement
AI marketing can send personalized emails, push notifications, and in-app messages automatically. This keeps customers engaged and informed.
Imagine this scenario: You have a loyal customer, David, who visits your ice cream parlor every Friday. You notice that he hasn’t been in for the past two Fridays. To prevent his churn, you could send him an automated email with a special “We Miss You” offer, perhaps a discount on his favorite ice cream flavor. This personalized gesture can rekindle his interest and bring him back.
Automation in customer engagement is like having a tireless assistant who sends out these messages at the perfect moment. It saves you time and ensures that no customer slips through the cracks.
Personalization at Scale
Imagine sending tailored offers to thousands of customers simultaneously. AI marketing makes it possible, increasing the chances of retaining customers.
In your ice cream parlor, personalization at scale would mean customizing offers for each customer’s unique preferences, even if you have hundreds of customers lining up. AI marketing algorithms analyze customer data in real-time, enabling you to send out mass personalized campaigns effortlessly.
For instance, on a scorching summer day, AI could identify all customers who have shown a preference for fruit-flavored ice creams. It would then send them a mass notification about a limited-time offer on refreshing fruit-flavored scoops. This kind of personalization grabs customers’ attention and keeps them loyal.
Real-time Decision Making with AI
AI marketing isn’t just about sending pre-scheduled messages. It can make real-time decisions based on customer behavior.
Imagine you have an online ice cream delivery service. A customer, Emily, visits your website and adds a tub of chocolate chip cookie dough ice cream to her cart. However, just before checking out, she hesitates. AI marketing can detect this hesitation and trigger a pop-up message offering a discount if she completes the purchase within the next five minutes. This real-time decision-making can turn a potential churn into a sale.
In the fast-paced world of customer churn, timing is everything. AI marketing ensures that you reach out to customers when it matters most, whether it’s to prevent churn or seize an upsell opportunity.
In summary, implementing AI marketing for churn prediction is like having a highly efficient and personalized marketing team that never sleeps. It seamlessly integrates with your CRM, automates customer engagement, scales personalization, and makes real-time decisions to keep your customers loyal and engaged.
Success Stories
How Netflix Reduced Churn with AI
Netflix, the streaming giant, faced a significant challenge – subscriber churn. To tackle this, they turned to AI and data analytics.
Netflix’s AI algorithms analyze vast amounts of data, including what users watch, when they watch, and for how long. They use this data to create personalized recommendations, making it more likely that users will find content they love. Additionally, Netflix uses AI to optimize the order of shows on their homepage, ensuring users see content that’s most relevant to them.
The result? A significant reduction in churn. Users are more likely to continue their subscriptions when they consistently find engaging content tailored to their preferences. Netflix’s use of AI is a prime example of how mastering churn prediction can have a substantial impact on a business’s bottom line.
The Starbucks Mobile App: A Lesson in Customer Retention
Starbucks, the global coffee giant, recognized the importance of customer retention early on. They developed a mobile app that not only facilitates payments but also offers a loyalty program and personalized recommendations.
The app tracks customer purchases and preferences. If a customer hasn’t visited a Starbucks store for a while, the app sends a notification with a tempting offer, like a free pastry with their next coffee purchase. This personalized approach keeps customers engaged and encourages them to return.
Starbucks’ success lies in its ability to use AI to predict when a customer is at risk of churning and take proactive measures to prevent it. Their app has become a blueprint for customer retention in the digital age.
Spotify’s Playlists: The Power of Personalization
Spotify, the music streaming service, has mastered the art of personalization. They use AI algorithms to curate playlists tailored to each user’s music taste.
Imagine you’re a Spotify user, and you enjoy listening to a mix of rock and pop. Spotify’s AI analyzes your listening history and creates a “Rock & Pop Favorites” playlist just for you. The more you listen, the better the recommendations become.
This level of personalization keeps users engaged with the platform. They don’t need to search for new music; Spotify brings it to their ears. As a result, Spotify has achieved impressive customer retention rates in the competitive music streaming industry.
Tesla’s Smart Car Features
Tesla, the electric car manufacturer, not only produces cutting-edge vehicles but also uses AI for predictive maintenance.
Tesla cars are equipped with sensors that continuously monitor various components, from battery health to tire pressure. When the AI detects an issue, it can alert the driver and schedule a service appointment automatically. This proactive approach not only enhances safety but also ensures customers’ vehicles remain in top condition.
Tesla’s use of AI not only prevents churn (as customers have fewer reasons to switch to other brands) but also fosters customer loyalty by providing a seamless and worry-free ownership experience.
These success stories underscore the power of AI in retaining customers and reducing churn. Whether it’s through personalized recommendations, proactive notifications, or predictive maintenance, AI has become a cornerstone of customer retention strategies.
The Future of AI Marketing and Churn Prediction
Predictive Analytics and Beyond
The future of AI marketing and churn prediction is incredibly exciting. Predictive analytics will become even more sophisticated, enabling businesses to anticipate churn with higher accuracy.
Imagine AI that not only predicts churn but also suggests tailored strategies to retain at-risk customers. It could recommend specific discounts, personalized content, or loyalty perks based on individual customer profiles.
Furthermore, AI will play a more prominent role in customer journey mapping. It will identify critical touchpoints where customers are most likely to churn and suggest interventions to keep them on the path to loyalty.
Ethical Considerations
As AI marketing advances, ethical considerations become paramount. Businesses will need to strike a delicate balance between personalization and privacy.
Imagine AI that knows more about you than you do – your preferences, habits, and even your emotions. While this can enhance the customer experience, it also raises concerns about data privacy and consent.
The future of AI marketing will involve stricter regulations and a heightened focus on transparent data practices. Businesses will need to prioritize customer trust to thrive in this landscape.
Staying Ahead in the AI Marketing Game
To stay competitive, businesses will need to invest in AI talent and technology. AI marketing will no longer be a luxury but a necessity.
Imagine a business landscape where AI marketing is as ubiquitous as social media. To stand out, companies will need to continuously innovate and push the boundaries of what AI can do. Staying ahead in the AI marketing game will become a strategic imperative.
AI’s Impact on Job Roles
AI marketing will reshape job roles in marketing and customer service. While some routine tasks will be automated, there will be a growing demand for AI specialists, data scientists, and AI ethicists.
Imagine a marketing department where AI collaborates with humans. AI takes care of data analysis, personalization, and automation, while humans provide creativity, strategy, and ethical oversight. This symbiotic relationship will define the workforce of the future.
In conclusion, the future of AI marketing and churn prediction promises a blend of technological advancement and ethical responsibility. As AI becomes more integrated into our daily lives, businesses that embrace it responsibly will thrive in a world where customer retention is the key to success.