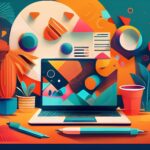
Achieving Data Harmony: BDatabase Integration Best Practices
December 14, 2023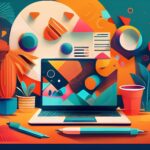
Strategic Account-Based Advertising: Reaching Decision Makers in Singapore and Asia
December 17, 2023The Evolution of ABM
Account-Based Marketing (ABM) has come a long way from its inception. Traditionally, marketers cast wide nets, hoping to catch as many leads as possible. ABM, however, shifts the focus from quantity to quality. It’s a strategic approach where marketing efforts are tailored to a select group of high-value accounts. This evolution stems from the need for more personalized and targeted marketing strategies.
Emergence of AI in Marketing
The emergence of Artificial Intelligence (AI) in marketing has added a new layer of sophistication to ABM. Gone are the days of manual segmentation and guesswork. AI introduces predictive analytics, automated decision-making, and machine learning, enabling marketers to make data-driven decisions with unprecedented precision. This section will explore how the integration of AI has become a catalyst for transforming traditional ABM strategies.
Understanding AI in ABM
Machine Learning in ABM
Machine learning, a subset of AI, plays a pivotal role in the success of ABM strategies. It involves the use of algorithms that analyze data, identify patterns, and make predictions. In the context of ABM, machine learning enables predictive lead scoring. By analyzing historical data, machine learning algorithms can identify high-value prospects, reducing the sales cycle length significantly. Moreover, it empowers marketers to make data-driven decisions, enhancing the overall efficiency of their strategies.
Natural Language Processing (NLP)
Natural Language Processing (NLP) takes center stage in redefining customer interactions within ABM. NLP allows for sentiment analysis, providing insights into how customers feel about products or services. Beyond sentiment analysis, NLP is leveraged in chatbots, creating dynamic and personalized customer interactions. Furthermore, it revolutionizes content creation by optimizing language and style based on audience preferences, ensuring maximum engagement.
Deep Learning for Personalization
Deep learning, a subset of machine learning, takes personalization in ABM to new heights. Dynamic content recommendations are a prime example. Deep learning algorithms analyze vast amounts of data, learning individual preferences, and tailoring content recommendations in real-time. This level of hyper-personalization not only boosts engagement but also creates a unique and memorable experience for the target audience.
Practical Applications of AI in ABM
Predictive Lead Scoring
Predictive lead scoring is a game-changer for marketers. Traditional lead scoring methods often rely on historical data and manual input. AI-driven predictive lead scoring, on the other hand, goes beyond, analyzing vast datasets to identify patterns that humans might overlook. By assigning scores based on the likelihood of conversion, marketers can prioritize high-value prospects, resulting in more efficient and targeted campaigns.
Intelligent Content Targeting
Content is king, but personalized content reigns supreme. AI enables intelligent content targeting by analyzing user behavior, preferences, and engagement patterns. Tailoring content for specific accounts ensures relevance and resonance, maximizing the impact of marketing efforts. Marketers can leverage AI insights to understand which type of content resonates most with different segments, refining their content strategy for optimal effectiveness.
Automated Email Campaigns
Email marketing remains a cornerstone of ABM strategies, and AI brings a new level of sophistication to this channel. Precision in email timing, driven by machine learning algorithms, ensures that emails reach prospects at the optimal moment. Dynamic content in emails, personalized based on user behavior and preferences, enhances engagement. A/B testing with AI insights allows marketers to refine their email campaigns continually, optimizing for the highest conversion rates.
AI-Enhanced Account Segmentation
AI-driven account segmentation goes beyond traditional demographic and firmographic factors. It dynamically adapts to changing customer behavior, ensuring that segments remain relevant over time. By analyzing real-time data, AI can identify emerging trends and shifts in customer preferences, allowing marketers to adjust their segmentation strategy accordingly. This flexibility is crucial in an ever-evolving market landscape.
Overcoming Challenges in AI-Driven ABM
Data Privacy and Security Concerns
As AI relies heavily on data, ensuring privacy and security is paramount. Marketers must navigate the complex landscape of data protection laws, with a particular focus on GDPR compliance. Safeguarding customer information is not only a legal requirement but also a trust-building exercise. This section will explore strategies for maintaining data integrity and building trust in AI-enabled ABM.
Integration with Existing Systems
The successful implementation of AI in ABM requires seamless integration with existing Customer Relationship Management (CRM) systems. This involves overcoming technological silos and ensuring that AI tools complement rather than disrupt existing workflows. Choosing the right AI solution that aligns with the organization’s technological infrastructure is critical for a smooth integration process.
Skill Gap and Training
Integrating AI into ABM strategies introduces a new set of skills that marketers need to acquire. Upskilling marketing teams is essential to harness the full potential of AI. Specialized training programs, both internal and external, can bridge the skill gap. However, addressing the human element in AI-driven ABM goes beyond technical skills; it involves fostering a culture that embraces innovation and adapts to change.
Budgetary Considerations
While the benefits of AI in ABM are substantial, there are budgetary considerations that marketers need to navigate. Calculating Return on Investment (ROI) in AI-driven ABM is crucial for justifying budget allocations. This section will explore how organizations can optimize their budget for AI implementation, ensuring long-term financial viability.
Future Trends: What Lies Ahead for AI in ABM
AI-Driven Predictive Customer Behavior Analysis
The future holds exciting possibilities with AI-driven predictive customer behavior analysis. Anticipating customer needs through advanced analytics and machine learning will redefine how marketers strategize, ensuring proactive and personalized approaches.
Blockchain Integration for Enhanced Security
As data privacy becomes more critical, the integration of blockchain in AI-driven ABM offers enhanced security. Transparent transactions and secure data sharing will build trust, creating a synergy between blockchain and AI in the realm of ABM.
Rise of AI-Powered Virtual Assistants
Virtual assistants powered by AI are on the horizon, transforming customer interactions. From answering queries to providing 24/7 support, these AI-driven virtual assistants will humanize the digital experience, making customer engagement more dynamic and personalized.
Conclusion
In this exploration of AI in ABM, we’ve journeyed through its evolution, dissected its components, and explored practical applications. The fusion of AI and ABM isn’t just a technological advancement; it’s a transformative force for marketers seeking unparalleled success. As we embrace the future trends, the synergy between AI and ABM will continue to redefine how we approach marketing strategies.