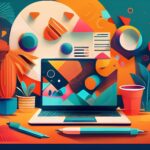
Cultivating Connections: The Power of Networking and Events in B2B Lead Generation
September 13, 2023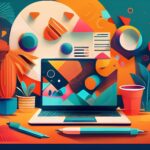
Crafting Compelling Lead Magnets: Turbocharging Your B2B Lead Generation Strategy
September 14, 2023What Are AI Marketing Recommendation Systems?
AI Marketing Recommendation Systems, often simply called recommendation systems or recommender systems, are sophisticated algorithms that analyze user data to provide personalized content, product recommendations, or suggestions. These systems are ubiquitous in our digital lives, from suggesting movies on Netflix to products on Amazon.
At their core, recommendation systems are designed to understand user preferences and behaviour. They leverage data such as past purchases, browsing history, and user interactions to make tailored suggestions. These suggestions can encompass a wide range of items, including products, services, articles, music, movies, and more. The ultimate goal is to enhance user experience, drive engagement, and increase conversion rates.
The Evolution of AI in Marketing
Before we delve into the intricacies of recommendation systems, let’s take a quick trip down memory lane. AI in marketing has come a long way, evolving from basic email personalization to the advanced systems we see today. The journey has been marked by significant advancements in machine learning, data analytics, and computing power.
In the early days of digital marketing, personalization was limited to addressing customers by their first names in email marketing campaigns. While this was a step in the right direction, it was far from the level of personalization we experience today. It was a time when marketing decisions were often based on intuition and broad demographic data.
As technology advanced, so did the capabilities of AI in marketing. Machine learning algorithms enabled marketers to analyze vast datasets and draw insights that were previously impossible to obtain manually. This shift towards data-driven decision-making paved the way for recommendation systems.
How Do Recommendation Systems Work?
Recommendation systems rely on two primary approaches: collaborative filtering and content-based filtering. These methods, often used in combination, enable recommendation systems to understand users’ tastes and preferences.
- Collaborative Filtering: This approach is based on the idea that users who have interacted with similar items in the past are likely to have similar preferences in the future. Collaborative filtering analyzes user behavior, such as item ratings and purchase history, to identify patterns and make recommendations. It can be further divided into user-based and item-based collaborative filtering, each with its strengths and weaknesses.
- Content-Based Filtering: Content-based filtering, on the other hand, focuses on the characteristics of items and user profiles. It recommends items that are similar to those the user has previously interacted with. For example, if a user frequently watches science fiction movies on a streaming platform, content-based filtering may recommend other science fiction titles.
Types of AI Marketing Recommendation Systems
Recommendation systems come in various flavors, each tailored to specific industries and use cases. Understanding the differences among these types is crucial for implementing an effective recommendation strategy.
- Collaborative Filtering: This type of recommendation system relies on the wisdom of the crowd. It analyzes user interactions with items and identifies patterns to make recommendations. It’s commonly used in e-commerce, streaming services, and social media platforms.
- Content-Based Filtering: Content-based recommendation systems focus on the attributes of items and user profiles. They recommend items similar to those the user has previously shown interest in. This is widely used in music and movie recommendations.
- Hybrid Systems: Hybrid recommendation systems combine collaborative and content-based filtering to provide more accurate and diverse recommendations. By leveraging both user behavior and item attributes, these systems offer a holistic approach to personalization.
- Matrix Factorization: Matrix factorization techniques break down user-item interaction data into latent factors, making it possible to uncover hidden patterns. This approach has found applications in recommendation systems for both products and content.
- Deep Learning: Deep learning models, particularly neural collaborative filtering, have gained popularity in recent years. These models use neural networks to capture complex relationships in user-item interactions, leading to highly accurate recommendations.
In summary, AI marketing recommendation systems are not one-size-fits-all solutions. The choice of the right recommendation approach depends on the nature of your business, the type of content or products you offer, and the quality of your data.
Benefits of Implementing AI Marketing Recommendations
Personalized Customer Experiences
Imagine entering a store where the shelves rearrange themselves based on your preferences. AI recommendation systems do just that in the digital realm. They curate content and products tailored to individual tastes, enhancing the overall customer experience.
Personalization is more than just addressing customers by their first names in emails; it’s about understanding their unique preferences and catering to them. When users feel that a platform understands their needs, they are more likely to engage and return.
Personalization extends beyond product recommendations. It can include tailoring website layouts, email content, and even pricing based on user behavior and preferences. For example, an e-commerce site might display sports equipment prominently to a customer who frequently browses sports-related items.
The benefits of personalized customer experiences are manifold:
- Higher Engagement: Personalized content captures users’ attention and keeps them engaged for longer periods.
- Increased Loyalty: When customers feel understood and catered to, they are more likely to remain loyal to a brand.
- Better Conversions: Tailored product recommendations can significantly boost conversion rates, leading to increased revenue.
- Enhanced User Satisfaction: Users appreciate platforms that save them time by presenting relevant options upfront.
- Implementing personalization through AI marketing recommendation systems is not just a trend; it’s becoming an expectation among consumers. Brands that invest in personalization are poised to gain a competitive edge and foster lasting customer relationships.
Enhanced Customer Engagement
Engagement is the lifeblood of customer loyalty. By recommending relevant and interesting content, recommendation systems keep customers engaged with your brand, increasing the likelihood of repeat interactions.
Consider the example of a streaming service like Netflix. When users log in, they are greeted with a curated list of movies and TV shows tailored to their tastes. This immediate personalization creates a sense of excitement and anticipation, making users more likely to click and start watching. The more time users spend engaged with the platform, the less likely they are to cancel their subscriptions.
The power of engagement through recommendations extends to various industries:
- E-commerce: By suggesting products related to a customer’s previous purchases, e-commerce platforms encourage users to explore additional items, ultimately leading to more sales.
- Social Media: Social networks use recommendation algorithms to curate users’ newsfeeds, ensuring they see posts and updates that are relevant to their interests and connections.
- News and Content Platforms: Recommendation systems play a crucial role in delivering personalized news articles, blogs, and videos, keeping users informed and engaged.
Enhanced engagement is not just about increasing the time users spend on a platform; it’s about creating a positive and memorable user experience. When users consistently find value in your recommendations, they are more likely to become loyal customers.
Improved Conversion Rates
Recommendation systems are masters at upselling and cross-selling. By suggesting complementary products or services, they can significantly boost conversion rates, ultimately increasing your revenue.
Let’s take the example of an e-commerce website. When a customer adds a camera to their shopping cart, the recommendation system can instantly suggest related items, such as camera lenses, tripods, or camera bags. If the customer finds these suggestions appealing, they are more likely to make additional purchases, increasing the average order value.
The impact of recommendation systems on conversion rates can be substantial:
- Higher Revenue: By encouraging customers to purchase related or complementary products, recommendation systems directly contribute to increased sales.
- Enhanced Customer Lifetime Value: Customers who regularly receive relevant recommendations are likely to become repeat buyers, leading to long-term revenue growth.
- Effective Inventory Management: Recommendation systems help clear out excess inventory by promoting products that might otherwise go unnoticed.
- Reduced Abandoned Carts: By engaging customers with enticing recommendations, recommendation systems can reduce cart abandonment rates.
It’s important to note that the effectiveness of recommendation systems in improving conversion rates is closely tied to their ability to understand user preferences accurately. The more data and insights the system has, the more targeted and persuasive its recommendations become.
Efficient Resource Allocation
Traditional marketing strategies often involve broad, one-size-fits-all campaigns. AI recommendations help allocate resources more efficiently by targeting specific customer segments with higher conversion potential. This not only saves resources but also enhances ROI.
Consider a scenario where a marketing team plans to run an email campaign promoting a new line of premium fitness equipment. Without the insights provided by a recommendation system, the team might send the same email to the entire subscriber list. While some recipients may be interested, many others might not have any interest in fitness equipment.
Now, let’s introduce AI recommendations into the equation. The recommendation system analyzes the past behavior of subscribers and segments them into groups based on their interests. It identifies a segment of subscribers who have previously purchased fitness-related products or shown interest in fitness content. The email campaign is then tailored specifically for this segment, featuring personalized product recommendations.
The benefits of efficient resource allocation through AI recommendations are evident:
- Higher ROI: By targeting the right audience with personalized content, marketing campaigns generate better returns on investment.
- Reduced Ad Spend Waste: Advertising budgets are optimized as ads are shown to users who are more likely to convert.
Improved Customer Satisfaction: Customers receive content and offers that align with their interests, leading to a more positive experience.
Efficient resource allocation is not limited to email marketing. It extends to various marketing channels, including display advertising, social media advertising, and website content. By ensuring that marketing efforts are directed where they are most likely to yield results, AI marketing recommendation systems optimize the use of resources.
In summary, the benefits of implementing AI marketing recommendation systems are far-reaching. They encompass personalized customer experiences, enhanced engagement, improved conversion rates, and efficient resource allocation. These advantages collectively contribute to building customer loyalty and driving business growth.
AI Marketing Recommendation Systems in Action
To understand the true power of AI marketing recommendation systems, let’s take a closer look at how they are revolutionizing various industries.
E-commerce: The Amazon Example
Amazon’s recommendation engine is a prime (pun intended) example of AI in action. By analyzing purchase history and browsing behavior, it suggests products that customers are likely to buy, contributing significantly to Amazon’s revenue.
When you visit Amazon, you’re greeted with a personalized homepage that showcases product recommendations tailored to your preferences. These recommendations are based on a combination of factors, including your past purchases, items you’ve added to your shopping cart, items you’ve rated, and even what other users with similar browsing patterns have purchased.
For example, if you recently bought a set of cookware, Amazon’s recommendation system might suggest kitchen gadgets, recipe books, or complementary items like utensils. By doing so, Amazon not only enhances your shopping experience but also encourages you to explore and purchase additional products.
The impact of recommendation systems on e-commerce is profound:
- Increased Sales: By showcasing relevant products, e-commerce platforms can significantly boost sales and revenue.
- Customer Retention: Users who receive personalized recommendations are more likely to return to the platform for future purchases.
- Inventory Optimization: Recommendation systems help clear slow-moving inventory by promoting products to interested customers.
- Reduced Cart Abandonment: By guiding customers to discover more products, recommendation systems can reduce cart abandonment rates.
Amazon’s success with recommendation systems serves as a testament to the effectiveness of AI-driven personalization in the e-commerce sector.
Streaming Services: Netflix’s Recommendation Engine
Netflix’s success is not solely attributed to its vast content library but also its recommendation system. Analyzing your viewing history and preferences keeps you hooked on personalized movie and TV show suggestions.
When you log in to Netflix, you’re presented with a curated list of titles, each selected based on your previous viewing behavior. If you’re a fan of suspenseful thrillers, you’ll likely see recommendations for movies and shows in that genre. Likewise, if you enjoy romantic comedies, Netflix will ensure that your homepage is populated with titles that match your tastes.
The brilliance of Netflix’s recommendation engine lies in its ability to cater to a diverse range of viewers. Whether you’re into documentaries, science fiction, or stand-up comedy, Netflix has personalized recommendations waiting for you. This level of personalization keeps users engaged, reduces churn, and encourages them to explore new content.
The impact of recommendation systems on streaming services is evident:
Viewer Satisfaction: Users find content that aligns with their interests quickly, leading to higher satisfaction.
- Reduced Churn: Personalized recommendations reduce the likelihood of subscribers cancelling their subscriptions.
- Discoverability: Recommendation systems introduce viewers to content they might not have found otherwise, broadening their content consumption.
- Netflix’s recommendation engine is a prime example of how AI can revolutionize the entertainment industry by delivering tailored content experiences to millions of subscribers worldwide.
Social Media: Facebook’s Personalized Feeds
Ever wondered why your Facebook feed is so addictive? It’s because of AI recommendation algorithms. They curate posts, ensuring you see content from friends and pages you engage with the most.
When you open Facebook, your newsfeed is populated with posts, articles, and videos from your friends and the pages you follow. But not every piece of content makes it to your feed. Facebook’s recommendation system assesses your interactions, such as likes, comments, and shares, to determine which content is most relevant to you.
For example, if you frequently interact with posts from a close friend or a particular news outlet, Facebook’s algorithm will prioritize showing you more content from those sources. Additionally, if you’ve shown interest in topics like travel, food, or technology, you’ll see posts related to those interests.
The effectiveness of Facebook’s recommendation system is evident:
- User Engagement: A personalized newsfeed keeps users engaged with the platform, leading to longer session durations.
- Content Relevance: Users see content that matters to them, increasing the likelihood of interactions and sharing.
- Platform Retention: Facebook’s recommendation engine plays a role in keeping users on the platform, reducing attrition.
Facebook’s success in retaining user engagement and time spent on the platform can be attributed, in part, to its AI-driven recommendation system.
Travel and Hospitality: Airbnb’s Dynamic Pricing
Airbnb employs recommendation systems not just for property suggestions but also for dynamic pricing. By considering factors like demand and availability, it optimizes prices, benefiting both hosts and guests.
Imagine you’re planning a weekend getaway and search for accommodations on Airbnb. You’ll notice that the prices for a particular listing can vary based on when you plan to stay. This variability is the result of Airbnb’s dynamic pricing recommendation system in action.
Airbnb’s recommendation engine analyzes various data points, including historical booking patterns, local events, and seasonal trends, to determine optimal pricing. For example, if there’s a music festival happening in the city you plan to visit, Airbnb may adjust prices for nearby listings to reflect increased demand.
The impact of recommendation systems on the travel and hospitality industry is significant:
- Optimized Pricing: Dynamic pricing recommendations ensure that hosts can maximize their earnings during peak demand periods.
- Enhanced User Experience: Guests benefit from competitive pricing and a wide range of accommodation options.
- Inventory Utilization: Airbnb’s recommendation system helps hosts efficiently utilize their properties, ensuring bookings even during off-peak periods.
Airbnb’s dynamic pricing recommendation system illustrates how AI can benefit both service providers and customers in the travel and hospitality sector.
In conclusion, AI marketing recommendation systems are not confined to a single industry or use case. They are reshaping the way businesses operate in e-commerce, streaming services, social media, and travel and hospitality. These systems enhance user experiences, drive engagement, and optimize business operations, all while contributing to customer loyalty.
Measuring the Impact on Customer Loyalty
Measuring the impact of AI marketing recommendation systems on customer loyalty is crucial for evaluating their effectiveness and making informed decisions. Here, we’ll explore key performance indicators (KPIs) for loyalty, the distinction between customer retention and acquisition, and provide case studies on loyalty enhancement.
Key Performance Indicators (KPIs) for Loyalty
To assess the impact on customer loyalty, businesses can monitor several KPIs:
- Customer Retention Rate: This KPI measures the percentage of customers who continue to engage with the brand over a specific period. An increase in retention rate indicates improved loyalty.
- Customer Lifetime Value (CLV): CLV quantifies the total value a customer brings to a business throughout their entire relationship. Higher CLV suggests stronger loyalty.
- Repeat Purchase Rate: This metric tracks the percentage of customers who make multiple purchases. It reflects ongoing engagement and loyalty.
- Net Promoter Score (NPS): NPS gauges customer satisfaction and the likelihood of recommending the brand to others. A higher NPS often correlates with higher loyalty.
- Churn Rate: The churn rate measures the percentage of customers who discontinue their relationship with the brand. A decrease in churn rate indicates improved loyalty.
- Time-to-Repeat Purchase: This metric evaluates how quickly customers make their second purchase. A shorter time frame suggests stronger loyalty.
- Customer Feedback and Surveys: Collecting qualitative data through customer feedback and surveys can provide valuable insights into loyalty drivers and areas for improvement.
Customer Retention vs. Acquisition
It’s essential to distinguish between customer retention and acquisition when assessing the impact of recommendation systems. Customer retention focuses on keeping existing customers engaged and loyal, while customer acquisition aims to attract new customers.
Recommendation systems primarily influence customer retention by enhancing user experiences, increasing engagement, and encouraging repeat purchases. While they can indirectly support acquisition by drawing in new customers through personalized recommendations, their primary role is in strengthening existing customer relationships.
Case Studies on Loyalty Enhancement
Let’s explore a few real-world case studies that demonstrate how recommendation systems have enhanced customer loyalty:
Amazon Prime
Amazon Prime, with its membership program, exemplifies the power of recommendation systems in driving loyalty. Prime members enjoy benefits such as free two-day shipping, access to Prime Video, and exclusive deals. Amazon’s recommendation engine plays a pivotal role in keeping Prime members engaged by suggesting products and content that align with their preferences. This, in turn, encourages Prime members to continue their subscriptions, contributing to Amazon’s impressive retention rates.
Spotify
Spotify’s recommendation system, powered by machine learning, is renowned for its ability to keep users engaged with personalized playlists. Discover Weekly, Release Radar, and Daily Mixes provide users with fresh music tailored to their tastes. By continually delivering music that resonates with users, Spotify fosters loyalty and keeps listeners returning to the platform.
Netflix
Netflix’s commitment to personalization extends beyond content recommendations. It applies recommendation algorithms to create personalized artwork and trailers for each show or movie. This level of customization not only enhances user engagement but also strengthens loyalty. Users feel that Netflix understands their preferences and caters to their viewing experience.
In conclusion, measuring the impact of AI marketing recommendation systems on customer loyalty involves tracking KPIs, understanding the distinction between retention and acquisition, and studying real-world case studies. These systems have a demonstrated track record of enhancing customer loyalty in various industries, making them valuable assets for businesses aiming to build lasting relationships with their customers.
Future Trends and Innovations
As AI marketing recommendation systems continue to evolve, several future trends and innovations are on the horizon. Let’s explore some of the exciting developments that will shape the landscape of recommendation technology.
AI-Generated Content
AI-generated content is poised to revolutionize the way businesses interact with customers. Recommendation systems will increasingly leverage AI-generated content to create personalized messages, product descriptions, and even articles. This hyper-personalization will not only enhance user experiences but also streamline content creation for businesses.
For instance, an AI recommendation system could analyze a customer’s browsing history and dynamically generate a product description that resonates with their preferences. This level of personalization can significantly impact engagement and conversion rates.
Voice and Conversational AI
Voice-activated recommendation systems are gaining prominence with the rise of virtual assistants like Amazon’s Alexa and Apple’s Siri. Users can now ask for personalized recommendations verbally, making the interaction more intuitive and natural.
Conversational AI will play a pivotal role in delivering recommendations through chatbots and virtual assistants. Customers can engage in real-time conversations, ask questions, and receive tailored suggestions. This trend will further enhance user engagement and provide businesses with valuable insights into customer preferences.
Augmented Reality (AR) Recommendations
Augmented reality is set to revolutionize the way customers experience products. Recommendation systems will leverage AR to enable customers to visualize how products fit into their lives before making a purchase.
For example, a furniture retailer could use AR to recommend sofas and chairs that match a customer’s room dimensions and decor style. Customers can use their smartphones or AR glasses to project virtual furniture into their living spaces, creating a highly immersive and personalized shopping experience.
Hyper-Personalization
The future of recommendation systems lies in hyper-personalization. These systems will not only consider individual preferences but also account for context and real-time data. For example, a recommendation system for a food delivery app might consider a user’s dietary preferences, location, time of day, and local restaurant availability to provide highly relevant meal suggestions.
Hyper-personalization will require advanced AI algorithms and access to vast datasets. However, the payoff in terms of customer loyalty and engagement will be substantial.
In conclusion, the future of AI marketing recommendation systems is exciting, with trends such as AI-generated content, voice and conversational AI, augmented reality recommendations, and hyper-personalization set to redefine customer experiences. Staying at the forefront of these innovations will be essential for businesses seeking to cultivate customer loyalty in a rapidly evolving digital landscape.