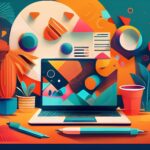
Enhancing Decision-Making with Predictive Analytics in 2024
June 28, 2024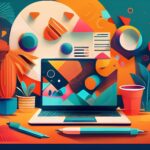
Optimizing SEO with AI: Strategies for Marketers in 2024
July 1, 2024The Importance of Audience Segmentation in Media Planning
Audience segmentation is a cornerstone of effective media planning, akin to serving customized meals to diners with diverse tastes and dietary preferences. In essence, it involves dividing a broad consumer base into smaller, more manageable groups based on shared characteristics such as demographics, behaviors, and preferences. This targeted approach ensures that marketing messages are relevant, engaging, and resonate with each specific group.
Imagine launching a campaign without segmentation—it’s like broadcasting a message to an anonymous crowd, hoping that some listeners will find it relevant. This scattergun approach is not only inefficient but also wasteful. In contrast, segmentation allows for precision targeting, ensuring that each message hits its mark. This leads to improved engagement, higher conversion rates, and a better return on investment (ROI). It’s about knowing your audience so well that your message feels like a one-on-one conversation rather than a generic broadcast.
Segmentation enables marketers to tailor their content to meet the specific needs and preferences of different audience groups. For instance, a beauty brand might segment its audience by age, offering anti-aging products to older consumers while promoting trendy makeup to younger ones. This personalized approach not only improves the effectiveness of the marketing campaign but also enhances the customer experience, fostering loyalty and long-term relationships.
In today’s digital age, where consumers are bombarded with countless marketing messages daily, relevance is key. Audience segmentation allows brands to cut through the noise and deliver messages that matter to their audience. This is particularly important in media planning, where the goal is to reach the right people at the right time with the right message. By understanding the unique characteristics of different audience segments, marketers can optimize their media spend and maximize the impact of their campaigns.
The Role of AI in Revolutionizing Audience Targeting
Enter Artificial Intelligence (AI), the game-changer in the realm of audience targeting. Traditional segmentation methods, while effective to a degree, are often limited by the sheer volume and complexity of data involved. They rely heavily on manual processes and human intuition, which can be time-consuming and prone to error. AI, on the other hand, leverages advanced algorithms and vast computing power to analyze large datasets quickly and accurately.
AI can process and analyze data from various sources—social media interactions, website analytics, purchase histories, and more—to identify patterns and trends that might not be apparent to human analysts. This enables a level of precision in audience targeting that was previously unimaginable. For example, AI can predict future behaviors based on past actions, allowing marketers to anticipate and respond to the needs of their audience proactively.
Moreover, AI-driven audience segmentation is dynamic and adaptive. Unlike traditional methods that often rely on static data, AI continuously learns and evolves as new data comes in. This means that the segmentation is always up-to-date, reflecting the latest trends and behaviors. This agility is crucial in today’s fast-paced digital landscape, where consumer preferences can change rapidly.
In addition to enhancing precision and efficiency, AI brings a new level of personalization to audience targeting. By understanding the individual preferences and behaviors of each segment, AI enables marketers to create hyper-personalized content and experiences. This not only boosts engagement and conversion rates but also builds stronger connections with the audience.
Brief Overview of What the Blog Will Cover
In this comprehensive guide, we will explore the fascinating world of AI-driven audience segmentation and its transformative impact on media planning. We will begin by defining key concepts and differentiating AI-driven segmentation from traditional methods. Next, we will delve into the key technologies that power AI segmentation, including machine learning algorithms, natural language processing, predictive analytics, and real-time data processing.
We will then outline the steps to implement AI-driven segmentation in your media planning strategy, from data collection and integration to continuous optimization and refinement. Following this, we will examine practical applications of AI segmentation in media planning, such as hyper-personalized content delivery, dynamic ad targeting, cross-channel campaign optimization, and customer journey mapping.
To bring these concepts to life, we will present case studies of successful AI-driven segmentation campaigns across different industries. We will also address common challenges in AI-driven segmentation and offer solutions for overcoming them. Finally, we will look ahead to future trends in AI-driven audience segmentation, including advanced predictive modeling, integration with emerging technologies, and ethical considerations.
By the end of this blog, you will have a thorough understanding of how AI-driven audience segmentation can enhance your media planning efforts and boost your campaign performance. Whether you are a seasoned marketer or a newcomer to the field, this guide will equip you with the knowledge and insights needed to harness the power of AI for your marketing strategy.
Understanding AI-Driven Audience Segmentation
Definition and Key Concepts
AI-driven audience segmentation is the practice of using artificial intelligence to analyze and categorize audience data into distinct segments based on various criteria. This approach goes beyond traditional segmentation methods by leveraging advanced algorithms to uncover deeper, more nuanced insights about the audience. The goal is to create highly targeted and personalized marketing strategies that resonate with specific groups of consumers.
At its core, AI-driven segmentation involves collecting vast amounts of data from various sources, such as social media interactions, purchase histories, website behavior, and more. This data is then processed using machine learning algorithms, which can identify patterns and correlations that may not be immediately obvious. For example, AI can determine that a particular segment of customers who frequently browse skincare products also tend to purchase health supplements, allowing marketers to target them with relevant offers.
One of the key concepts in AI-driven segmentation is the idea of dynamic segmentation. Unlike traditional methods that often rely on static data, AI-driven segmentation is continuously updated as new data comes in. This ensures that the segments are always current and relevant, reflecting the latest trends and behaviors. This dynamic nature is particularly valuable in fast-changing markets, where consumer preferences can shift rapidly.
Another important concept is the granularity of segmentation. Traditional methods might group consumers into broad categories based on a few key characteristics, such as age or income. AI, however, can analyze a multitude of factors simultaneously, creating more detailed and precise segments. For instance, instead of just targeting “millennials,” AI can identify sub-segments such as “tech-savvy millennials interested in eco-friendly products” or “millennial parents looking for educational toys.”
How AI Differs from Traditional Segmentation Methods
Traditional segmentation methods often rely on demographic data and simple statistical techniques to group consumers. While this approach can provide some useful insights, it is limited by the quality and scope of the data. Manual analysis can be time-consuming and prone to error, and the resulting segments are often broad and static.
AI-driven segmentation, on the other hand, utilizes advanced algorithms and machine learning techniques to analyze vast datasets quickly and accurately. This allows for more precise and dynamic segmentation. AI can process data from a variety of sources, including structured data like purchase histories and unstructured data like social media posts. This holistic approach provides a more comprehensive view of the audience.
Furthermore, AI-driven segmentation can uncover hidden patterns and correlations that might be missed by traditional methods. For example, AI might identify that customers who frequently engage with a brand’s social media posts are also more likely to make purchases during holiday sales. These insights enable marketers to create more targeted and effective campaigns.
The use of predictive analytics is another key differentiator. Traditional methods often rely on historical data to create segments, but AI can use this data to predict future behaviors. This means that marketers can anticipate the needs and preferences of their audience and tailor their strategies accordingly. For example, AI can predict which customers are most likely to respond to a particular promotion, allowing marketers to target them more effectively.
Benefits of AI-Driven Segmentation for Media Planning
The benefits of AI-driven segmentation for media planning are manifold. First and foremost, it enhances the precision and effectiveness of marketing campaigns. By understanding the unique characteristics and preferences of different audience segments, marketers can create personalized content that resonates with each group. This leads to higher engagement rates and improved conversion rates.
AI-driven segmentation also improves efficiency. Traditional segmentation methods can be labor-intensive and time-consuming, requiring manual data analysis and interpretation. AI automates these processes, allowing marketers to focus on strategy and creativity rather than data crunching. This not only saves time but also reduces the risk of human error.
Another significant benefit is the ability to adapt to changing consumer behaviors. Because AI-driven segmentation is dynamic and continuously updated, it can quickly respond to new data and trends. This agility ensures that marketing strategies remain relevant and effective, even in rapidly changing markets.
Moreover, AI-driven segmentation can provide deeper insights into audience behaviors and preferences. By analyzing a wide range of data points, AI can uncover hidden patterns and correlations, offering a more comprehensive understanding of the audience. This enables marketers to make more informed decisions and develop more targeted strategies.
Finally, AI-driven segmentation can enhance ROI by optimizing media spend. By targeting the right audience with the right message at the right time, marketers can maximize the impact of their campaigns and minimize waste. This not only improves the effectiveness of marketing efforts but also ensures that resources are used more efficiently.
Key Technologies Powering AI Audience Segmentation
Machine Learning Algorithms
At the heart of AI-driven audience segmentation are machine learning algorithms. These algorithms are designed to analyze large datasets and identify patterns and relationships within the data. There are several types of machine learning algorithms used in audience segmentation, each with its own strengths and applications.
Supervised Learning: In supervised learning, the algorithm is trained on a labeled dataset, meaning that the input data comes with the correct output. For example, if the task is to segment customers based on their purchasing behavior, the algorithm is trained on historical data where the customer segments are already known. The algorithm learns the relationship between the input features (such as purchase history, demographic information, etc.) and the output labels (the segments). Once trained, the algorithm can predict the segments of new, unseen customers based on their input features.
Unsupervised Learning: Unsupervised learning algorithms, on the other hand, are used when the data does not come with predefined labels. These algorithms attempt to find the underlying structure in the data by clustering or grouping similar data points together. In the context of audience segmentation, unsupervised learning can be used to identify natural segments within a customer base based on similarities in their behavior or characteristics. For example, a retailer might use unsupervised learning to discover that their customers can be grouped into distinct segments based on their shopping patterns, even if they were not previously aware of these segments.
Reinforcement Learning: Reinforcement learning is a type of machine learning where the algorithm learns by interacting with its environment and receiving feedback in the form of rewards or penalties. This approach is particularly useful for dynamic and evolving datasets, as the algorithm continuously learns and adapts based on the outcomes of its actions. In audience segmentation, reinforcement learning can be used to optimize marketing strategies in real time, adjusting the segmentation and targeting based on the performance of different campaigns.
Natural Language Processing (NLP)
Natural Language Processing (NLP) is a branch of AI that focuses on the interaction between computers and human language. NLP enables AI to understand, interpret, and generate human language, making it crucial for analyzing textual data such as social media posts, customer reviews, and other forms of user-generated content.
In the context of audience segmentation, NLP can be used to analyze and categorize textual data to understand the sentiment, preferences, and behaviors of different audience segments. For example, NLP can analyze social media conversations to identify common themes and topics that resonate with different segments. This allows marketers to create content that is tailored to the specific interests and preferences of each segment.
NLP can also be used to analyze customer feedback and reviews to identify areas for improvement and opportunities for engagement. By understanding the sentiment behind the words, NLP can provide valuable insights into how customers feel about a brand and its products. This information can be used to enhance customer experience and build stronger relationships with different audience segments.
Predictive Analytics
Predictive analytics uses historical data and statistical algorithms to predict future outcomes. In audience segmentation, predictive analytics can forecast how different segments are likely to respond to various marketing strategies. By understanding these potential outcomes, marketers can tailor their campaigns to maximize effectiveness.
For example, predictive analytics can be used to identify which customers are most likely to make a purchase based on their past behavior. This allows marketers to target these high-potential customers with personalized offers and promotions. Predictive analytics can also identify emerging trends and shifts in consumer behavior, enabling marketers to stay ahead of the curve and adapt their strategies accordingly.
Real-Time Data Processing
Real-time data processing is the ability to analyze and process data as it is generated. This capability is essential for dynamic and adaptive audience segmentation. By processing data in real time, AI can quickly adjust segmentation strategies in response to new information.
For example, an e-commerce site can use real-time data processing to analyze a customer’s browsing behavior and deliver personalized product recommendations instantly. This immediate response enhances the customer experience and increases the likelihood of conversion.
Real-time data processing also enables marketers to monitor the performance of their campaigns and make adjustments on the fly. This agility ensures that marketing efforts are always based on the most current data, increasing their relevance and impact.
Steps to Implement AI-Driven Audience Segmentation
Data Collection and Integration
The first step in implementing AI-driven audience segmentation is data collection. This involves gathering data from various sources, such as social media, website analytics, customer databases, and third-party providers. The quality and comprehensiveness of the data are crucial, as they form the foundation of the AI models.
Data collection should be as exhaustive as possible, capturing a wide range of attributes that can provide insights into consumer behavior. For example, demographic data, such as age, gender, and income, can provide a basic understanding of who the audience is. Behavioral data, such as browsing history, purchase history, and social media interactions, can provide insights into what the audience does and how they engage with the brand.
Once the data is collected, it needs to be integrated into a unified system. This can involve data cleaning, transformation, and normalization to ensure consistency and accuracy. Data cleaning involves removing any errors or inconsistencies in the data, such as duplicate records or missing values. Data transformation involves converting the data into a format that is suitable for analysis, such as standardizing units of measurement or encoding categorical variables. Data normalization involves scaling the data to ensure that all attributes are on a similar scale, which is important for many machine learning algorithms.
AI Model Selection and Training
Selecting the right AI model is critical for effective audience segmentation. There are various models to choose from, depending on the nature of the data and the specific goals of the segmentation. For example, if the goal is to predict future behavior, a predictive model such as a decision tree or a neural network might be appropriate. If the goal is to identify natural segments within the data, a clustering model such as k-means or hierarchical clustering might be more suitable.
Once a model is selected, it needs to be trained using historical data. This training process involves feeding the model data and allowing it to learn patterns and relationships. The model’s performance is then evaluated and fine-tuned to optimize its accuracy and efficiency. This can involve adjusting the model’s parameters, selecting different features, or using different training algorithms.
Segment Identification and Analysis
After the AI model is trained, it can start identifying and analyzing audience segments. This involves grouping individuals based on shared characteristics and behaviors. The AI can uncover segments that may not be immediately obvious, providing deeper insights into the audience.
These segments can then be analyzed to understand their unique traits and preferences. For example, a segment might be identified as frequent buyers who prefer online shopping and are highly responsive to email marketing. Another segment might be occasional buyers who prefer in-store shopping and are more influenced by social media. This detailed understanding allows marketers to create targeted strategies for each segment.
Continuous Optimization and Refinement
AI-driven segmentation is not a one-time process; it requires continuous optimization and refinement. As new data is collected, the AI model needs to be updated and retrained to ensure it remains accurate and relevant. This ongoing process allows marketers to adapt to changing audience behaviors and preferences, maintaining the effectiveness of their campaigns over time.
Continuous optimization also involves monitoring the performance of the segmentation strategy and making adjustments as needed. This can involve testing different marketing messages, channels, and tactics to see what works best for each segment. By continuously learning and adapting, marketers can ensure that their strategies remain effective and relevant in a dynamic and ever-changing market.
Applications of AI Segmentation in Media Planning
Hyper-Personalized Content Delivery
One of the most exciting applications of AI-driven segmentation is hyper-personalized content delivery. By understanding the specific preferences and behaviors of different segments, marketers can create and deliver content that is tailored to individual needs. This level of personalization enhances user engagement and satisfaction, leading to higher conversion rates.
For example, an e-commerce site can use AI to recommend products based on a user’s past purchases and browsing history. If a customer frequently buys running shoes and sportswear, the AI can suggest new arrivals in athletic gear or exclusive offers on running accessories. This personalized approach not only makes the shopping experience more enjoyable but also increases the likelihood of repeat purchases.
Hyper-personalized content delivery is not limited to product recommendations. It can also be applied to other forms of content, such as emails, social media posts, and advertisements. By tailoring the content to the preferences and behaviors of each segment, marketers can create more engaging and relevant experiences. For example, a travel company can send personalized travel itineraries and offers based on a customer’s past trips and preferences.
Dynamic Ad Targeting
Dynamic ad targeting uses AI to deliver ads that are tailored to the specific interests and behaviors of different audience segments. This ensures that ads are relevant and engaging, increasing the likelihood of a positive response. AI can also optimize ad placement in real time, adjusting strategies based on performance data. This dynamic approach maximizes the effectiveness of ad campaigns and improves ROI.
For example, an online retailer can use AI to target ads for winter coats to customers who live in colder climates and have shown an interest in winter clothing. The AI can also adjust the ad targeting based on real-time data, such as weather conditions or current trends. If a sudden cold snap hits, the AI can increase the frequency of winter coat ads to maximize their impact.
Dynamic ad targeting is particularly effective for cross-channel campaigns. AI can analyze how different segments interact with different channels, such as social media, search engines, and display networks. By understanding these interactions, AI can optimize ad placements to ensure that each segment sees the most relevant ads on the most effective channels.
Cross-Channel Campaign Optimization
AI-driven segmentation enables cross-channel campaign optimization, ensuring a consistent and cohesive experience across various platforms. By understanding how different segments interact with different channels, marketers can tailor their strategies to maximize engagement. For example, a segment that prefers social media can be targeted with tailored ads on Facebook and Instagram, while another segment that engages more with email can receive personalized email campaigns.
Cross-channel optimization is not just about targeting the right channels; it’s also about creating a seamless and integrated experience for the audience. AI can analyze the entire customer journey, from initial awareness to final purchase, and identify the most effective touchpoints for each segment. This allows marketers to create a cohesive and consistent experience that guides the customer through the funnel.
For example, a fashion retailer can use AI to analyze how different segments interact with their website, social media, and email campaigns. By understanding these interactions, the retailer can create a cohesive and personalized experience for each segment. For example, a customer who frequently engages with social media posts can be targeted with personalized ads and offers on social media, while a customer who prefers email can receive tailored email campaigns with exclusive discounts and promotions.
Customer Journey Mapping
Customer journey mapping involves tracking and understanding the various touchpoints a customer interacts with before making a purchase. AI-driven segmentation enhances this process by providing deeper insights into the behaviors and preferences of different segments. This allows marketers to create more effective and personalized customer journeys, improving the overall customer experience.
AI can analyze data from various sources to understand how different segments navigate the customer journey. For example, it can identify which touchpoints are most important for each segment, such as product reviews, social media interactions, or email campaigns. This information can be used to create personalized and seamless experiences that guide customers through the journey.
Customer journey mapping is particularly valuable for identifying pain points and opportunities for improvement. For example, if a segment frequently abandons their shopping carts at the checkout stage, AI can identify the reasons behind this behavior and suggest strategies to address it. This might involve simplifying the checkout process, offering incentives, or providing additional support.
By understanding the unique characteristics and preferences of different segments, AI-driven customer journey mapping enables marketers to create more effective and personalized experiences. This not only improves customer satisfaction and loyalty but also increases the likelihood of conversion and repeat purchases.
Future Trends in AI-Driven Audience Segmentation
Advanced Predictive Modeling
As AI technology continues to evolve, we can expect to see more advanced predictive modeling techniques being applied to audience segmentation. These techniques will enable marketers to forecast future behaviors and trends with greater accuracy, allowing them to proactively adapt their strategies.
For example, advanced predictive modeling can identify emerging segments and predict how they will respond to different marketing strategies. This enables marketers to stay ahead of the curve and create more effective and targeted campaigns. Additionally, predictive modeling can help identify at-risk segments and develop strategies to retain and engage them.
Integration with Emerging Technologies (e.g., IoT, 5G)
The integration of AI-driven segmentation with emerging technologies such as IoT and 5G will open up new possibilities for personalization and targeting. IoT devices generate vast amounts of data, providing deeper insights into consumer behaviors and preferences. For example, smart home devices can provide data on how consumers interact with different products and services, enabling more personalized and relevant marketing.
5G technology, with its high-speed connectivity and low latency, will enable real-time data processing and analytics, enhancing the effectiveness of AI-driven segmentation. This will allow marketers to deliver personalized and dynamic content and ads in real time, based on the latest data and trends.
Ethical AI and Responsible Targeting
As AI-driven segmentation becomes more prevalent, ethical considerations and responsible targeting will become increasingly important. Companies must ensure that their AI-driven strategies are transparent, fair, and respectful of consumer privacy.
One key aspect of ethical AI is avoiding bias in AI models. This requires careful selection and preparation of training data, as well as regular auditing and testing of AI models to identify and address any biases. Additionally, companies should adopt responsible targeting practices, ensuring that their marketing strategies are respectful and considerate of consumer preferences and privacy.
Conclusion
Recap of Key Benefits
AI-driven audience segmentation offers numerous benefits for media planning. It enhances precision and effectiveness, allowing marketers to create personalized and relevant experiences for different audience segments. It improves efficiency by automating data analysis and optimization processes, and it enables dynamic and adaptive strategies that can respond to changing consumer behaviors and preferences. By leveraging AI-driven segmentation, companies can improve engagement, increase conversions, and achieve a higher ROI.
The Future of Media Planning with AI Segmentation
The future of media planning lies in the continued advancement and integration of AI-driven segmentation. As AI technology evolves, we can expect to see more sophisticated and accurate segmentation techniques, as well as new possibilities for personalization and targeting. By embracing these advancements and addressing the challenges associated with AI-driven segmentation, companies can unlock the full potential of AI and achieve greater success in their marketing efforts.
Call to Action for Embracing AI in Media Planning
To stay competitive in the dynamic and ever-changing market, companies must embrace AI-driven segmentation and incorporate it into their media planning strategies. This requires investment in the right technologies and infrastructure, as well as a commitment to data privacy and ethical AI practices. By leveraging the power of AI-driven segmentation, companies can create more effective, personalized, and engaging experiences for their audiences, driving growth and success in the digital age.