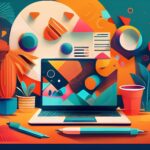
Unlocking the Future of SEO: How AI Marketing Transforms Voice Search Strategies
August 29, 2023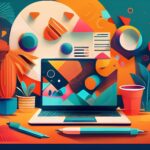
AI’s Game-Changing Impact: Personalized Content Generation in Marketing Revolution
August 30, 2023Integrating artificial intelligence (AI) has brought remarkable advancements in the ever-evolving digital marketing landscape. AI-driven marketing tactics have revolutionized how businesses reach and engage with their target audiences. However, with great power comes great responsibility, and as AI marketing tactics become more sophisticated, so do fraudulent practices attempting to exploit them. This blog post dives deep into the realm of advanced fraud detection, uncovering the techniques and strategies to fortify AI marketing tactics against deceptive practices.
Understanding AI in Marketing
The Marriage of AI and Marketing
Artificial intelligence and marketing have merged to create a synergistic alliance that wields data-driven insights like never before. AI’s prowess in processing colossal datasets and identifying intricate patterns has transformed marketing into a realm of precision. It enables businesses to forecast consumer behavior, personalize interactions, and make strategic decisions with unprecedented accuracy. The amalgamation of AI and marketing isn’t just a partnership; it’s a revolution altering the customer engagement landscape.
AI-Powered Personalization: A Game Changer
Imagine receiving product recommendations that resonate with your unique preferences before realizing them. AI-powered personalization is rewriting the rules of customer engagement. By analyzing historical data, AI predicts individual preferences, enabling businesses to tailor their offerings in ways previously thought impossible. This level of personalization creates a profound connection between brands and customers, enhancing loyalty and driving sales. In essence, AI transforms businesses from mere providers to intuitive problem solvers.
Rising Threats: AI-Enabled Fraud
With the rise of AI in marketing comes an alarming uptick in AI-driven fraud. Fraudsters are capitalizing on the sophistication of AI to craft deceptive strategies that mimic genuine interactions. From AI-generated phishing emails to deepfakes impersonating high-profile figures, the line between authentic and fabricated is blurring. The rapid evolution of AI-enabled fraud poses a significant challenge that demands innovative solutions. As AI reshapes marketing, it’s simultaneously shaping the dark underbelly of fraudulent practices.
The Cat and Mouse Game: Fraud vs. Detection
Evolving Face of Digital Fraud
Fraud has transcended its traditional forms and morphed into a digital juggernaut armed with AI. Deepfakes, automated bots, and AI-generated content have become tools of deception. Social engineering attacks have taken on a new dimension as AI refines the art of manipulation. As businesses embrace AI for marketing, fraudsters quickly exploit its potential for malicious intent, requiring an arms race in fraud detection.
Unmasking Deceptive Practices
Deceptive practices are no longer confined to glaring red flags; they’ve evolved into sophisticated maneuvers that often slip under the radar. Advanced fraud detection hinges on identifying subtle deviations from established patterns. It demands an acute understanding of user behavior and a willingness to scrutinize anomalies that might go unnoticed. The challenge lies in creating algorithms distinguishing creative marketing tactics and malicious fraud.
The Crucial Role of Advanced Detection Systems
As AI fortifies the arsenal of fraudsters, it simultaneously equips defenders with advanced detection systems. These systems leverage AI’s analysis and pattern recognition prowess to identify anomalous behavior. Machine learning algorithms tirelessly learn from historical data, enabling them to evolve alongside new fraud tactics. The effectiveness of these systems hinges on their ability to adapt swiftly to emerging threats and counteract them with precision.
Advanced Techniques in Fraud Detection
Behavioral Analysis and Anomaly Detection
Unveiling fraud often begins with understanding how genuine users interact with digital platforms. Behavioral analysis establishes baselines of typical behavior, enabling the detection of deviations or anomalies. For instance, sudden transaction frequency or location changes can raise red flags, prompting further investigation.
Machine Learning Models as Guardians
Machine learning models, the unsung heroes of fraud detection, excel at spotting patterns that evade human eyes. These models identify correlations and anomalies indicative of fraud by processing vast datasets. Their adaptive nature means they can evolve to recognize new types of fraud as they emerge.
Natural Language Processing (NLP) in Uncovering Trickery
Fraudulent communication often hides behind a veneer of authenticity. NLP dissects language patterns, contextual cues, and sentiment to distinguish between legitimate and deceitful content. A digital lie detector scrutinizes our words to unearth hidden motives.
Network Analysis: Mapping the Fraud Ecosystem
Fraud operates in networks, with different actors playing distinct roles. Network analysis unveils these connections, helping investigators understand the underlying structure of fraudulent activities. By mapping out relationships, it becomes easier to disrupt and dismantle these networks.
Strategies to Safeguard AI Marketing
Data Security: The Foundation of Trust
In the battle against fraud, data integrity is the linchpin. Safeguarding customer data through secure storage, encryption, and access controls is crucial for maintaining trust. A breach compromises customer trust and provides fraudsters with the ammunition they need.
Continuous Monitoring and Adaptive Learning
Fraud detection is a dynamic process that requires continuous vigilance. Advanced systems not only monitor real-time activities but also learn from new data. This adaptive learning ensures that detection mechanisms evolve with evolving fraud tactics.
Human-AI Collaboration: Two Heads Are Better Than One
The human intuition for spotting irregularities pairs harmoniously with AI’s analytical rigor. Collaborative efforts between humans and AI bring together the strengths of both worlds, resulting in more comprehensive and effective fraud detection.
Ethical Implications and Transparency
As AI infiltrates fraud detection, ethical considerations come to the forefront. Striking a balance between privacy and security is imperative. Transparency in disclosing the role of AI in fraud detection is pivotal for maintaining customer trust.
Real-Life Cases: Learning from the Past
The battle between fraudulent practices and cutting-edge fraud detection techniques is far from theoretical—it’s a real-world conflict with significant consequences. By examining past instances where deceptive practices exploited AI marketing tactics, we gain valuable insights into the methods employed by fraudsters and the lessons we can glean from these experiences.
The Instagram Influence Scam
In social media marketing, influencer collaborations have become the norm. However, fraudsters saw an opportunity to exploit the trust that influencers build with their followers. The Instagram Influence Scam involved the creation of fake influencer accounts, complete with a convincing follower count and engagement metrics. These impostors reached out to brands for collaborations, promising a broad reach. Unsuspecting brands fell victim to this scam, believing they were partnering with influential figures when, in reality, they were pouring resources into fraudulent campaigns.
Impact: Brands wasted marketing budgets on fake collaborations and suffered reputational damage when customers discovered they were misled. The scam highlighted the importance of verifying the authenticity of influencers and the need for AI-powered tools to identify anomalies in engagement patterns and follower behavior.
Lessons Learned: AI-powered fraud detection platforms can scrutinize engagement metrics and follower patterns to distinguish between authentic and fake influencers. These platforms can identify sudden spikes in followers or unusual engagement patterns by analyzing historical data, helping brands make informed decisions about potential collaborations.
Deepfake Dilemmas in Video Marketing
Video marketing is a powerful tool for engaging audiences, but deepfake technology introduced a new layer of complexity. Deepfakes are AI-generated videos that convincingly depict individuals saying or doing things they never actually did. In one instance, fraudsters used deepfake technology to create videos of prominent figures endorsing a product or service. These videos spread rapidly on social media, leading consumers to believe these endorsements were genuine.
Impact: The spread of deepfake videos eroded trust in video marketing and directly impacted brand reputations. Consumers questioned the authenticity of endorsements and became skeptical of videos, even those with legitimate messages.
Lessons Learned: To combat deepfake-driven misinformation, AI tools specializing in image and video analysis are crucial. These tools can assess the authenticity of videos by examining facial expressions, lip movements, and inconsistencies that might indicate manipulation. They play a vital role in ensuring that video marketing remains a credible and effective strategy.
Chatbot Conundrums: Bypassing AI Defenses
Chatbots have transformed customer interactions, but they’ve also become a target for fraudsters. In a chatbot conundrum, fraudsters manipulated chatbots into divulging sensitive information or facilitating unauthorized transactions. By exploiting vulnerabilities in chatbot programming, fraudsters crafted conversational paths that led to fraudulent outcomes.
Impact: Consumers were lured into divulging personal information or making financial transactions under the false belief that they were interacting with legitimate entities. The problem highlighted the need for enhanced security measures to protect businesses and customers.
Lessons Learned: AI-driven chatbot security relies on continuous monitoring and adaptive learning. Businesses can prevent fraudsters from exploiting vulnerabilities by analyzing chatbot interactions and identifying suspicious patterns. Incorporating natural language processing and machine learning into chatbot design enhances their ability to detect and respond to potentially fraudulent interactions.
Future-Proofing Your AI Marketing Approach
The digital landscape is a dynamic, ever-shifting terrain, and future-proofing your AI marketing approach is not just a strategy—it’s a necessity. As AI continues its rapid evolution, fraudsters will adapt their tactics accordingly. Here’s how you can prepare for the challenges of tomorrow.
Zero-Day Fraud Attack Preparedness
Zero-day attacks exploit vulnerabilities that have just been discovered or made public, giving businesses “zero days” to prepare. Future-proofing against such attacks involves staying up-to-date with the latest fraud trends and continuously improving your detection and response strategies.
Consider the example of a financial institution that proactively monitors the dark web for chatter about potential zero-day attacks targeting customer accounts. By detecting these discussions early, they can implement countermeasures before the attack gains momentum.
Blockchain’s Role in Authentication
Blockchain, the technology underpinning cryptocurrencies, is finding new applications beyond digital currencies. Its decentralized and tamper-proof nature makes it a formidable tool in authentication and verification. Future AI marketing approaches should consider integrating blockchain to ensure data integrity, transaction transparency, and user identity protection.
Imagine an e-commerce platform that utilizes blockchain to authenticate the origin of products and their journey from manufacturer to consumer. This builds trust and thwarts fraudsters attempting to introduce counterfeit goods into the market.
AI Ethics Committees: Shaping the Landscape
As AI becomes more intertwined with our lives, ethical considerations gain prominence. Future-proofing your AI marketing approach involves establishing AI ethics committees within your organization. These committees can help shape responsible AI usage, establish guidelines for data privacy, and ensure that AI-driven practices align with ethical standards.
A tech company could form an AI ethics committee comprising data scientists, legal experts, and representatives from different departments. This committee would review AI algorithms to ensure they don’t inadvertently reinforce biases or compromise user privacy.
Collaborative Resilience: Industry Partnerships
Fraud knows no boundaries, and tackling it requires a united front. Collaborative efforts among industry players, governments, and private sectors can fortify defenses and make the digital landscape safer.
Knowledge Sharing Among Competitors
In a surprising twist, competitors can become allies in the fight against fraud. Industry players often face similar challenges, and sharing insights about emerging fraud tactics can help everyone stay ahead of the curve. This collective knowledge can be instrumental in identifying new threats and developing effective countermeasures.
Imagine a consortium of e-commerce giants sharing anonymized data about fraudulent transactions. By pooling their resources, they can identify trends and patterns that individual companies might miss.
Government and Private Sector Collaboration
Fraud is a multifaceted challenge requiring a combined effort from the public and private sectors. Governments can enact regulations that provide a legal framework for combating fraud, while private sector entities can contribute by sharing expertise and data.
Consider a scenario where a government agency partners with technology companies to create a centralized platform for reporting and analyzing fraud incidents. This collaboration accelerates incident response and enhances overall security.
Global Efforts to Combat AI-Driven Fraud
The fight against AI-driven fraud transcends national borders. International cooperation is vital to curb cross-border fraud networks. Global initiatives that facilitate information exchange and joint efforts can yield a more coordinated response to emerging threats.
Imagine an international consortium of cybersecurity organizations working to identify and mitigate AI-driven fraud targeting financial institutions across different countries. By sharing threat intelligence, they create a web of protection that covers a broader scope.
AI Tools for Fraud Detection
The arms race between fraudsters and those defending against them has led to the development of powerful AI tools dedicated to fraud detection. These tools leverage the same technological advancements that fuel fraudulent activities but direct them toward safeguarding digital landscapes. Let’s explore some of the cutting-edge AI tools that are redefining the field of fraud detection.
AI-Driven Fraud Detection Platforms
Dedicated AI platforms are emerging as central hubs for fraud detection efforts. These platforms combine the strengths of machine learning, data analysis, and pattern recognition to identify anomalies in real-time data streams. These platforms can swiftly detect deviations from normal behavior by analyzing historical data and continuously learning from new information.
An example of such a platform is “FraudGuard AI,” which employs a combination of predictive modeling, behavioral analytics, and rule-based systems to detect fraud across various industries. The platform uses machine learning algorithms to adapt to evolving fraud tactics, ensuring higher detection accuracy and lower false favorable rates.
Cognitive Computing for Pattern Recognition
Cognitive computing surpasses traditional algorithms by mimicking the human brain’s cognitive abilities. This advanced form of AI can recognize complex patterns and detect anomalies that might elude rule-based systems. Cognitive computing is beneficial in detecting sophisticated fraud schemes that involve multiple interconnected events.
“CogniDetect,” an AI-powered solution, stands out in this arena. It employs cognitive computing to analyze data across different touchpoints, uncovering hidden patterns and connections that signify fraudulent activities. By understanding the context of data points, it can identify even the most subtle fraud indicators.
Predictive Analytics for Anticipating Threats
Predictive analytics leverages historical data to make informed predictions about future events. When applied to fraud detection, it can foresee potential threats based on patterns exhibited by past fraudulent activities. This proactive approach allows businesses to take preventive measures before fraud occurs.
“FraudForecast” is a prime example of a predictive analytics tool for fraud detection. It uses machine learning algorithms to analyze historical data, identify trends, and predict the likelihood of specific fraud scenarios. This empowers businesses to allocate resources strategically to mitigate potential risks.
Automated Decision-Making in Real Time
Fraud detection often requires split-second decision-making to prevent ongoing fraudulent activities. AI tools that offer real-time decision-making capabilities are invaluable in this context. These tools can autonomously assess incoming data streams, classify events as usual or suspicious, and trigger appropriate actions.
“RealTimeGuard” exemplifies this category of AI tools. It employs real-time data processing and machine learning to make instant decisions about the legitimacy of transactions or interactions. This swift response is essential for preventing financial losses and minimizing the impact of fraudulent activities.
The need for advanced fraud detection has become paramount in the ever-evolving dance between AI marketing tactics and fraudulent practices. As technology progresses, so does the ingenuity of those who seek to exploit it. By understanding the marriage of AI and marketing, unmasking deceptive practices, and leveraging advanced detection techniques, businesses can fortify their AI-driven strategies against fraudulent assaults. Collaboration, innovation, and ethical considerations will shape the landscape, ensuring AI remains a force for good in marketing. With knowledge as the ultimate armor, businesses can confidently navigate the intricate dance floor of AI marketing while keeping fraudsters at bay.
FAQs
- How does AI impact fraud detection in marketing? AI revolutionizes fraud detection by analyzing vast datasets, identifying patterns, and detecting anomalies often invisible to humans. It equips businesses with advanced tools to stay ahead of evolving fraud tactics.
- What role does behavioral analysis play in fraud detection? Behavioral analysis establishes standard user behavior patterns, allowing systems to identify deviations that might indicate fraudulent activities—sudden changes in behavior, such as irregular transaction locations, trigger alerts for further investigation.
- Can AI-powered fraud detection systems adapt to new fraud tactics? Yes, AI-powered fraud detection systems employ adaptive learning. They continuously update their algorithms based on new data, ensuring they can identify and counteract emerging fraud tactics.
- How does blockchain enhance fraud detection? Blockchain’s immutable ledger provides a tamper-proof record of transactions and interactions, adding an extra layer of trust to authentication processes and making it harder for fraudsters to manipulate data.
- Why is collaboration crucial in combating AI-driven fraud? Fraud is a global issue that transcends industry boundaries. Collaborative efforts among competitors, governments, and the private sector facilitate knowledge sharing and the development of more effective strategies against fraud.